Stack your deck with an AI education
AI may seem as mystifying as a magic trick, but a peek behind the curtain will reveal its secrets.
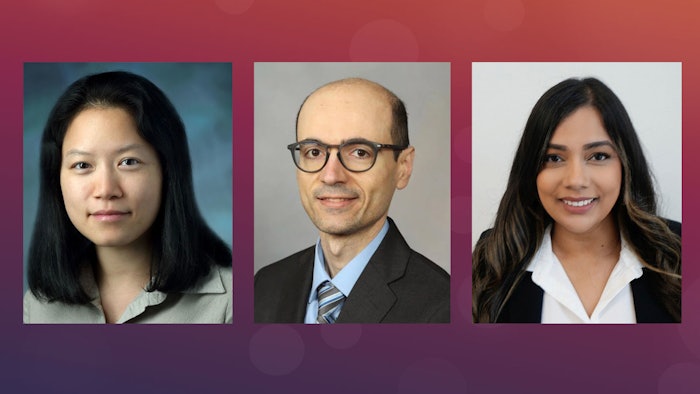
The term “artificial intelligence” is becoming more ubiquitous in hospital settings every day. But what does it really mean? And how will it impact your day-to-day life as a hospitalist?
According to Gigi Liu, MD, MSc, hospitalist at the Johns Hopkins University School of Medicine, AI is already transforming hospital medicine by improving patient care, optimizing workflows, and assisting clinicians with complex decision making.
“AI-powered tools assist hospitalists by analyzing vast amounts of patient data to identify early warning signs of conditions like sepsis, acute kidney injury, or cardiac deterioration,” she said. “AI models trained on electronic health records, imaging, and lab results can detect patterns and provide risk stratifications, leading to earlier and more accurate diagnoses.”
And that’s just the tip of the iceberg. In Thursday’s session, “Rolling the Dice With AI: Mastering ‘AI Lingo’ During This Healthcare Revolution,” Dr. Liu, along with Sharmila Tilak, MD, research fellow at Brigham and Women’s Hospital in Boston, and Meltiady Issa, MD, MBA, FACP, SFHM, hospitalist at Mayo Clinic Rochester, will provide hands-on simulations with group activities using decks of cards and dice to demonstrate the processes involved in each subgroup of AI.
But first, the trio will provide an overview of those AI subgroups, including supervised learning, unsupervised learning, neural networks, and generative AI.
The differences between supervised and unsupervised learning can be confusing, said Dr. Tilak. Supervised learning is a type of machine learning in which a model is trained using labeled data.
“Think of it like a clinician teaching a medical student how to diagnose pneumonia using chest X-rays,” she said. “The student is given many X-rays along with the correct diagnosis (labeled pneumonia or no pneumonia). Over time, the student learns to recognize patterns that distinguish pneumonia from a normal lung.
With unsupervised learning, the model finds patterns in data without labeled examples.
“Unlike supervised learning where an algorithm learns from labeled data, unsupervised learning works more like a researcher exploring unknown conditions and looking for patterns,” Dr. Tilak said. “Imagine a clinician analyzing a large dataset of patient symptoms, lab values, and imaging without predefined diagnoses. The goal is to group similar patients together based on shared characteristics, potentially identifying unknown disease subtypes or predicting different risk profiles.”
Although AI can be a tremendous help to the medical community, Dr. Issa said it is not without its potential pitfalls. The top three pitfalls, he said, are hallucination and misinformation, bias and equity concerns, and ethical and legal risks.
AI models can “hallucinate” or generate false information that appears highly credible, which is particularly risky in hospital medicine where accuracy is critical.
“For example, if an AI-generated clinical summary fabricates a non-existent study or recommends an incorrect medication dose, it could lead to serious patient harm,” he said.
AI models also learn from historical medical data, which may contain biases related to race, gender, socioeconomic status, and geography. If the training data is skewed, Dr. Issa said AI could reinforce existing disparities in healthcare.
“For instance, an AI model trained mostly on data from urban hospitals might not perform well for rural or underserved populations,” he said. “This can lead to inaccurate diagnoses or suboptimal treatment recommendations for certain patient groups.”
But that’s not to say these potential pitfalls can’t be overcome. Dr. Liu said it will require hospitalists to play an active role in managing how AI is used in their environment. And the key to that is education.
“We, as hospitalists, should play an active role in learning to review and verify AI-generated outputs, ensuring fairness and diverse representation of datasets for the AI models, and creating clear AI governance policies, regulatory oversight, and clinician accountability,” she said. “In order to perform these tasks, we as hospitalists must have a basic understanding of how these various AI models work to anticipate and come up with solutions for mitigation.”
Visit SHM Meeting News Central for more coverage.